The financial industry is experiencing a groundbreaking transformation, thanks to the rapid advancements in Deep Learning. This revolutionary branch of Artificial Intelligence (AI) has paved the way for remarkable innovations, reshaping the way we handle money. Deep Learning algorithms, inspired by the human brain’s neural networks, have proven to be incredibly powerful in analyzing vast amounts of data and making accurate predictions. Know the various ways in which Deep Learning is transforming the finance industry, from revolutionizing investment strategies to enhancing fraud detection systems and improving customer experience.
Best Ways Of Deep Learning That Transforming the Finance Industry
The application of Deep Learning in the finance industry has resulted in numerous significant changes. Know the key areas where this technology is making a remarkable impact.
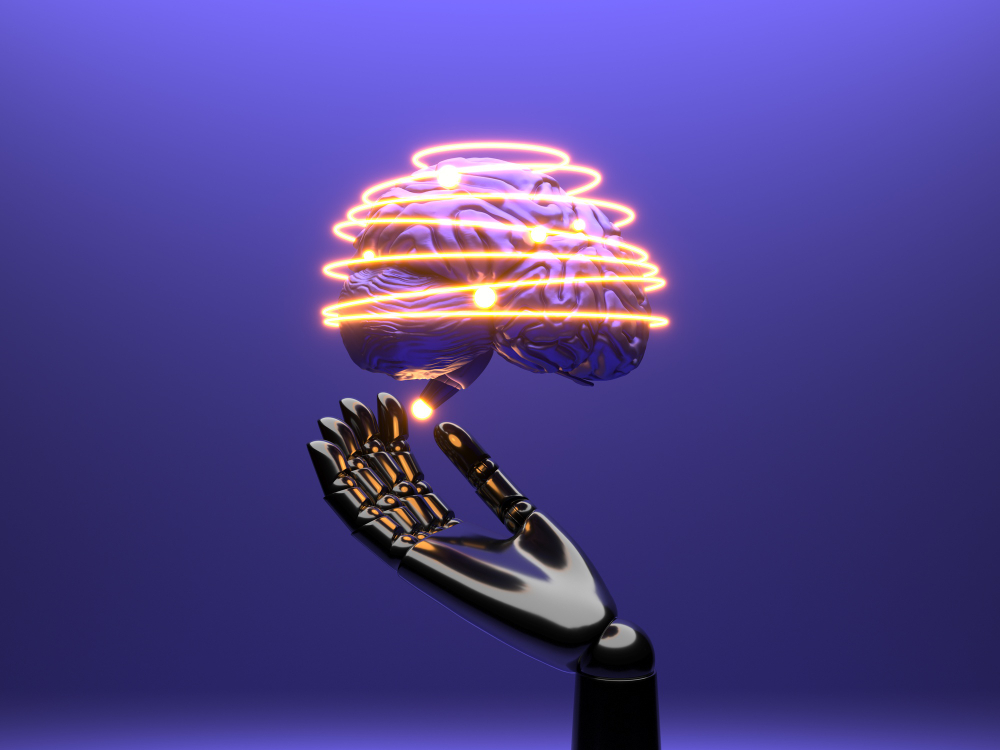
1. Transforming Investment Strategies
Deep Learning algorithms are revolutionizing investment strategies by enabling more accurate predictions and enhanced decision-making processes. Financial institutions can leverage these algorithms to analyze complex market data, identify patterns, and make data-driven investment decisions. By utilizing Deep Learning, investment firms can gain a competitive edge by making informed choices based on real-time market trends and historical data.
2. Improving Fraud Detection Systems
Deep Learning has become a game-changer in the fight against financial fraud. Traditional fraud detection systems often struggle to keep up with the evolving tactics of fraudsters. Deep Learning algorithms can analyze vast volumes of transactional data and detect anomalies with remarkable precision. By leveraging the power of Deep Learning, financial institutions can identify fraudulent activities in real time, preventing substantial losses and protecting their customers’ assets.
3. Transforming Customer Experience in Next Level
In the era of digital banking, customer experience is paramount. Deep Learning algorithms enable financial institutions to gain valuable insights into customer behavior and preferences, leading to highly personalized services. By analyzing customer data, such as transaction history and browsing patterns, Deep Learning algorithms can offer tailored recommendations, customized investment plans, and personalized financial advice. This level of personalization enhances customer satisfaction and strengthens customer loyalty.
4. Optimizing Risk Assessment
Deep Learning algorithms have also transformed the way financial institutions assess risks. Traditionally, risk assessment models relied on limited variables and simplistic calculations. With Deep Learning, institutions can analyze vast amounts of data, including historical financial data, market trends, and external factors, to generate more accurate risk assessments. This advanced risk assessment allows financial institutions to make better-informed decisions, reducing the chances of unexpected losses.
5. Streamlining Regulatory Compliance
Complying with regulatory requirements is a top priority for financial institutions. Deep Learning algorithms can automate compliance processes by analyzing and interpreting complex regulatory texts, identifying potential violations, and ensuring adherence to the necessary guidelines. By leveraging Deep Learning, financial institutions can streamline regulatory compliance, saving time, reducing errors, and minimizing the risk of non-compliance penalties.
6. Improving Loan Underwriting
Deep Learning has significantly improved the efficiency and accuracy of loan underwriting processes. By analyzing vast amounts of financial and non-financial data, such as credit history, employment records, and social media profiles, Deep Learning algorithms can assess borrowers’ creditworthiness more effectively. This enhanced underwriting process helps financial institutions make better lending decisions, reducing the risk of default and improving the overall loan portfolio quality.
Frequently Asked Questions (FAQs)
1. How does Deep Learning differ from traditional machine learning methods?
Deep Learning differs from traditional machine learning methods by employing multiple layers of artificial neural networks to process data. Unlike traditional machine learning algorithms, Deep Learning algorithms can automatically learn hierarchical representations of data, allowing for more complex and abstract pattern recognition.
2. Is Deep Learning only applicable to large financial institutions?
No, Deep Learning is applicable to financial institutions of all sizes. While large institutions may have more resources to invest in Deep Learning research and development, smaller institutions can still benefit by adopting pre-existing Deep Learning solutions or partnering with technology providers specializing in the finance industry.
3. What are the ethical considerations surrounding the use of Deep Learning in finance?
The use of Deep Learning in finance raises ethical considerations, particularly regarding data privacy and security. Financial institutions must ensure that customer data is adequately protected and used responsibly. transparency in algorithmic decision-making is crucial to prevent potential biases and maintain public trust.
4. Can Deep Learning completely eliminate fraud in the finance industry?
While Deep Learning algorithms can greatly enhance fraud detection systems, it is impossible to completely eliminate fraud. Fraudsters continually adapt their techniques, necessitating ongoing development and improvement of Deep Learning models. Deep Learning can significantly reduce fraud incidents and minimize financial losses.
5. Are there any limitations to Deep Learning in the finance industry?
Deep Learning in the finance industry has its limitations. The accuracy and reliability of Deep Learning models heavily depend on the quality and quantity of data available. Interpreting the decisions made by Deep Learning algorithms can be challenging, potentially raising concerns in terms of transparency and accountability.
6. How can financial professionals adapt to the integration of Deep Learning?
To adapt to the integration of Deep Learning, financial professionals should embrace continuous learning and upskilling. Understanding the capabilities and limitations of Deep Learning algorithms is crucial for leveraging their potential effectively. Collaboration with data scientists and AI experts can also help bridge the knowledge gap and drive successful implementation.
Conclusion
Deep Learning is transforming the finance industry by revolutionizing investment strategies, enhancing fraud detection systems, improving customer experience, optimizing risk assessment, streamlining regulatory compliance, and refining loan underwriting processes. As this cutting-edge technology continues to evolve, financial institutions must embrace the opportunities it presents while carefully addressing the associated challenges. By harnessing the power of Deep Learning, the finance industry can pave the way for a future that is more efficient, secure, and customer-centric
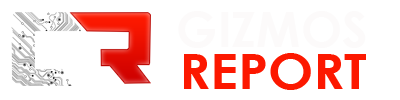